Realizing autonomous driving is one of the most challenging tasks in Artificial Intelligence research. The solution lies in building a new Turing test system which is able to test and verify the autonomous vehicle’s capability in understanding complex traffic scenario and making driving decision, and thus stimulate the development of autonomous driving technology.
How to test the system intelligence is a vital research issue in Artificial Intelligence. Based on Turing, safe and reliable AI systems can be achieved if and only if the tests have clear definitions of tasks and efficient methods to generate abundant data for tests.
After more than a decade painstaking work, a new self-driven closed-loop parallel testing system was finally built by researchers from Institute of Automation, Chinese Academy of Sciences (CASIA), Tsinghua University, and Xi’an Jiaotong University. The system focuses on implementing more challenging tests to accelerate the building and testing of autonomous vehicles. Related result, titled Parallel Testing of Vehicle Intelligence via Virtual-Real Interaction, was published as Focus in Artificial Intelligence on Science Robotics. Authors from CASIA made leading and important contributions to the research, and was acknowledged as joint first author and corresponding author in the work.
The research group constructed an intelligent closed-loop parallel testing system with a recognition mechanism to realize self-upgrading under human experts’ guidance. There are three parts in this system. The first part establishes a set of semantic definitions to characterize the tasks that should be finished by autonomous vehicles. The second part implements the tests for specified task instances. The field test and simulation test are tightly integrated into a parallel testing system which keeps collecting new field data to update the simulation system. The third part evaluates both vehicle performances and task difficulties to seek the most challenging new tasks.
Specially, an adversarial learning model was designed to automatically generate new task instances that may be harder than existing task instances based on the past testing results, aiming to push the autonomous vehicles to improve its capability in adjusting to complex environment. It is believed that the system can effectively accelerate the progress of autonomous driving technology.
The work was supported by National Natural Science Foundation of China.
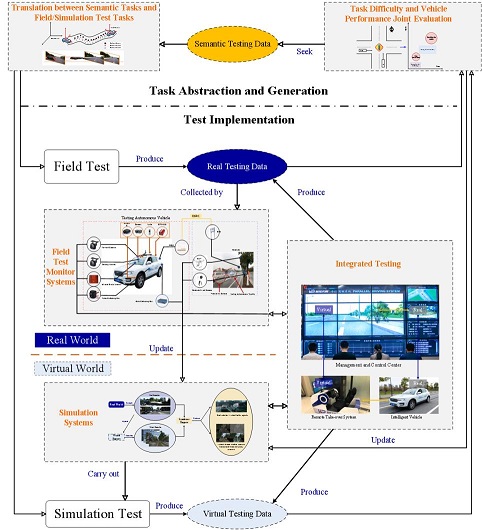
Full Text:
http://robotics.sciencemag.org/content/4/28/eaaw4106
Contact:
ZHANG Xiaohan, Institute of Automation, Chinese Academy of Sciences
Email: casia_en@ia.ac.cn